How AI is transforming asset management strategies
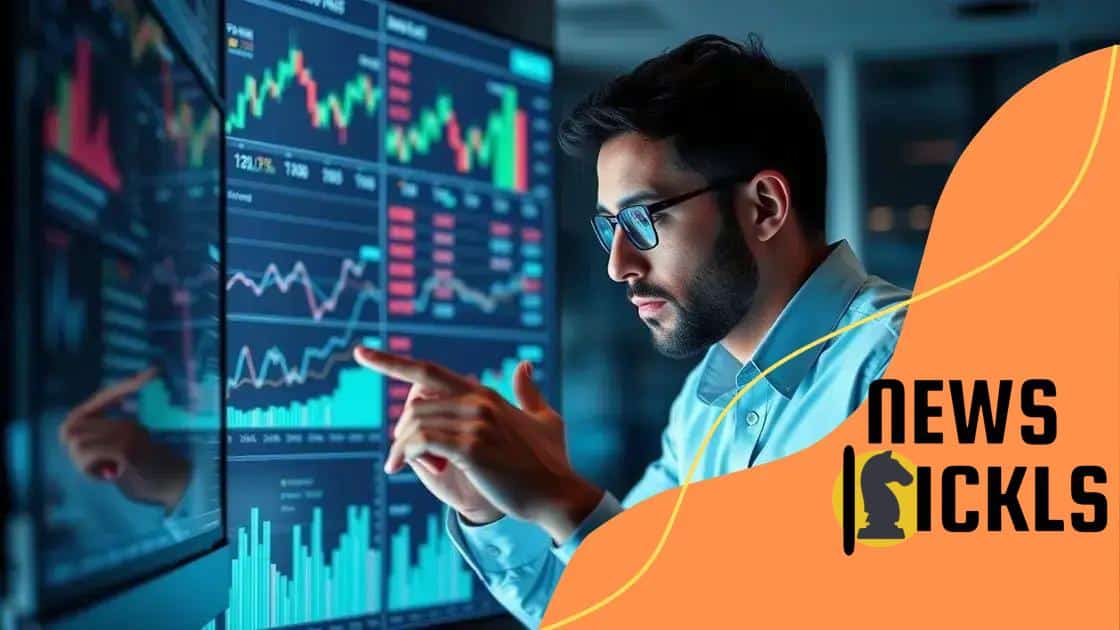
AI is transforming asset management strategies by enhancing decision-making, improving risk assessment, and optimizing portfolio management through data-driven insights and machine learning algorithms.
How AI is transforming asset management strategies is a game changer for financial firms today. It raises an interesting question: How can these technologies redefine traditional investment methods? Let’s dive in.
The role of AI in risk assessment
In today’s fast-paced financial world, the role of AI in risk assessment has become crucial. With its ability to analyze vast amounts of data quickly, AI assists firms in making informed decisions regarding investments and operations.
Understanding Risk Assessment
Risk assessment involves identifying potential hazards that could impact a company’s financial health. To effectively manage these risks, having a reliable system is essential. Here is where AI plays a significant role.
How AI Enhances Risk Evaluation
AI systems can process data more efficiently than humans, leading to quicker analysis and response times. By utilizing algorithms and machine learning, AI can identify patterns in data that may signal a potential risk.
- Data integration: AI gathers information from multiple sources, such as market trends and historical data.
- Predictive analytics: AI utilizes past performance to predict future outcomes.
- Continuous monitoring: Unlike traditional methods, AI enables real-time risk evaluation.
The continuous monitoring capabilities of AI allow firms to stay ahead of potential risks. These systems provide alerts for any significant changes or trends, empowering companies to make swift adjustments to their strategies.
The Advantages of AI in Risk Management
The integration of AI into risk management offers numerous benefits. First, it reduces manual errors, providing a more accurate assessment of risks. Second, the cost efficiency achieved through automation saves companies both time and money.
As firms continue to adopt AI technologies, the landscape of risk assessment will evolve. Leveraging AI not only improves the accuracy of evaluations but also helps firms respond to uncertainties swiftly and confidently.
Enhancing decision-making with AI
Enhancing decision-making with AI has become a significant focus for businesses aiming to improve their operational efficiency. With the ability to analyze data rapidly, AI empowers decision-makers with actionable insights.
The Importance of Data-Driven Decisions
In today’s environment, relying on intuition alone is not enough. Data-driven decisions ensure that companies make informed choices based on solid evidence rather than hunches.
AI-Powered Insights
AI can process massive datasets, uncovering trends that may be invisible to human analysts. By evaluating historical data, AI models can predict future outcomes reliably.
- Improved accuracy: AI’s analytical capabilities lead to more precise forecasts.
- Faster analysis: Decisions can be made quickly, responding to market changes.
- Reduced biases: AI minimizes human biases that often cloud judgment.
This shift toward AI-driven decision-making means businesses can react faster and with greater confidence. For instance, companies can optimize their inventory management by analyzing buying patterns and adjusting stock levels accordingly.
Real-World Applications
Many industries are already benefiting from AI in decision-making. For example, financial services use AI to assess credit risks and predict market movements, while healthcare providers utilize AI for patient diagnosis and treatment plans.
As companies continue to integrate AI tools, the landscape of decision-making will evolve. Embracing these technologies will lead to smarter, data-informed strategies, positioning businesses for success in a competitive environment.
AI-driven portfolio management techniques
AI-driven portfolio management techniques are reshaping how investors approach asset allocation and risk management. By leveraging artificial intelligence, portfolio managers can optimize their strategies based on real-time data.
Understanding AI’s Role in Portfolio Management
AI systems analyze vast amounts of market data, uncovering patterns and insights that human managers may overlook. This capability allows for more informed investment decisions.
Key Techniques in AI-Driven Management
There are several key techniques that AI employs in portfolio management. Here are some notable methods:
- Algorithmic trading: AI algorithms execute trades at high speeds based on preset criteria.
- Risk assessment models: AI assesses risks by analyzing market trends and historical data.
- Dynamic asset allocation: AI reallocates assets in real-time based on changing market conditions.
- Sentiment analysis: AI examines news articles and social media to gauge market sentiment.
These techniques enhance traditional portfolio management by providing tools for predictive analytics, allowing managers to forecast performance more accurately. As a result, investors can fine-tune their portfolios for improved returns and reduced risks.
The Future of AI in Portfolio Management
The future of portfolio management lies in continued advancements in AI technology. As these systems evolve, they will offer even more sophisticated methodologies for both institutional and individual investors. The increased integration of AI tools promises to democratize investing, making advanced strategies accessible to a wider audience.
The impact of machine learning on asset allocation
The impact of machine learning on asset allocation is profound. By harnessing data-driven insights, financial institutions are enhancing their approaches to investing.
Revolutionizing Asset Allocation
Machine learning algorithms analyze vast datasets quickly, identifying patterns that help in making informed investment choices. This technology enables investors to tailor their portfolios more effectively.
Key Benefits of Machine Learning in Asset Allocation
Investors gain numerous advantages when integrating machine learning into their strategies. Here are some key benefits:
- Enhanced forecasting: Machine learning improves the accuracy of predictions regarding asset performance.
- Real-time analysis: Algorithms can assess market conditions as they change, allowing for immediate adjustments.
- Personalization: Machine learning algorithms can create custom portfolios based on individual investor goals and risk tolerances.
- Risk management: Better insights into potential risks help investors safeguard their portfolios.
By using historical data, these algorithms can predict how different assets might perform under various market scenarios. This capability allows managers to optimize their asset allocations based on anticipated returns and risks.
Real-World Applications in Different Industries
In finance, machine learning is used for developing complex models that guide investment strategies. For instance, hedge funds utilize machine learning to analyze market trends, enabling them to make more strategic allocation decisions.
Overall, the introduction of machine learning is changing the asset allocation landscape. As it continues to evolve, asset managers will likely find even more innovative ways to leverage this technology for better investment outcomes.
Real-world examples of AI in asset management
Real-world examples of AI in asset management showcase how financial institutions are leveraging technology to enhance performance and efficiency. Many firms are already seeing the benefits of integrating artificial intelligence into their operations.
Case Study: BlackRock
BlackRock, one of the world’s largest asset management firms, uses AI to enhance its investment strategies. By employing algorithms to analyze market data, they can identify trends and optimize portfolio management. This integration helps them provide better service to clients and make data-driven decisions.
Case Study: UBS
UBS has also embraced AI to improve research and risk analysis. Their AI tools sift through massive datasets to provide insights that inform investment strategies. By doing so, they can respond more quickly to market changes and manage risks effectively.
Case Study: State Street
State Street has developed AI and machine learning applications to automate routine tasks and enhance operational efficiency. By using AI, they reduce manual errors and speed up processes, allowing their team to focus on more complex investment decisions.
Case Study: J.P. Morgan
J.P. Morgan employs AI for trade execution and market analysis. Their AI-driven trading tools help optimize execution strategies, improve performance, and enhance risk management.
These examples highlight how widespread and effective AI has become in the asset management industry. By leveraging technology, firms are not only improving their operational efficiency but also reallocating resources towards strategic decision-making.
In conclusion, the integration of AI into asset management is revolutionizing how companies operate. By leveraging AI technologies, firms can enhance decision-making, improve risk assessment, and optimize portfolio management. As seen in real-world examples, companies like BlackRock and J.P. Morgan are leading the way in adopting these innovations. Ultimately, AI not only provides better insights but also allows asset managers to focus on strategic elements that drive value for investors. As the technology continues to advance, we can expect even greater transformations in the asset management landscape.
FAQ – Frequently Asked Questions about AI in Asset Management
How does AI improve decision-making in asset management?
AI analyzes vast amounts of data quickly, providing insights that help portfolio managers make informed investment choices.
What are some real-world examples of AI in asset management?
Companies like BlackRock and J.P. Morgan use AI for risk assessment, portfolio optimization, and to enhance trading strategies.
Can AI help with risk assessment?
Yes, AI identifies potential risks by analyzing historical data and market trends, enabling firms to manage risks more effectively.
What benefits can asset managers gain from machine learning?
Machine learning provides enhanced forecasting, real-time analysis, and personalized investment strategies, improving overall portfolio performance.